Adversarial Knowledge Transfer from Unlabeled Data
In ACM International Conference on Multimedia (ACM-MM), 2020
Akash Gupta, Rameswar Panda, Sujoy Paul, Jianming Zhang, Amit K. Roy-Chowdhury
July 2020
Abstract
While machine learning approaches to visual recognition offer great promise, most of the existing methods rely heavily on the availability of large quantities of labeled training data. However, in the vast majority of real-world settings, manually collecting such large labeled datasets is infeasible due to the cost of labeling data or the paucity of data in a given domain. In this paper, we present a novel Adversarial Knowledge Transfer (AKT) framework for transferring knowledge from internet-scale unlabeled data to improve the performance of a classifier on a given visual recognition task. The proposed adversarial learning framework aligns the feature space of the unlabeled source data with the labeled target data such that the target classifier can be used to predict pseudo labels on the source data. An important novel aspect of our method is that the unlabeled source data can be of different classes from those of the labeled target data. Extensive experiments well demonstrate that models learned using our approach hold a lot of promise across a variety of visual recognition tasks on multiple standard datasets.
Publication
In ACM International Conference on Multimedia (ACM-MM), 2020
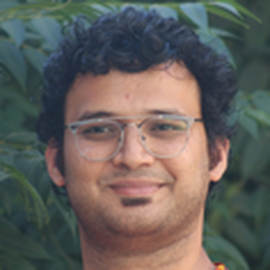
Senior Machine Learning Scientist
My research interests include computer vision amd machine learning applications in object detection and video enhancement.