Deep Quantized Representation for Enhanced Reconstruction
In IEEE International Symposium on Biomedical Imaging (ISBI Workshop), 2020
Akash Gupta, Abhishek Aich, Amit K. Roy-Chowdhury
January 2020
Abstract
While machine learning approaches have shown remarkable performance in biomedical image analysis, most of these methods rely on high-quality and accurate imaging data. However, collecting such data requires intensive and careful manual effort. One of the major challenges in imaging the Shoot Apical Meristem (SAM) of Arabidopsis thaliana, is that the deeper slices in the $z-$stack suffer from different perpetual quality related problems like poor contrast and blurring. These quality related issues often lead to the disposal of the painstakingly collected data with little to no control on quality while collecting the data. Therefore, it becomes necessary to employ and design techniques that can enhance the images to make them more suitable for further analysis. In this paper, we propose a data-driven Deep Quantized Latent Representation (DQLR) methodology for high-quality image reconstruction in the Shoot Apical Meristem (SAM) of Arabidopsis thaliana. Our proposed framework utilizes multiple consecutive slices in the $z$-stack to learn a low dimensional latent space, quantize it and subsequently perform reconstruction using the quantized representation to obtain sharper images. Experiments on a publicly available dataset validate our methodology showing promising results.
Publication
In IEEE International Symposium on Biomedical Imaging (ISBI Workshop), 2020
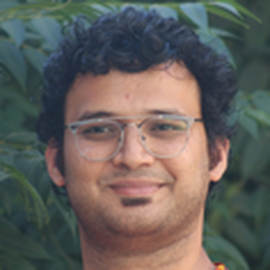
Senior Machine Learning Scientist
My research interests include computer vision amd machine learning applications in object detection and video enhancement.